Socio-Demographic Data Guide for Program Evaluation
Introduction and Scope
Advancing equity, diversity and inclusion is one of six core research and innovation values at the University of Toronto (U of T). Access to, and appropriate use of, actionable socio-demographic data is critical to advancing equity, diversity and inclusion efforts. An understanding of students’ and staff backgrounds can help programs improve access and students’ and staff experiences, success and overall sense of belonging.
Institutionally, the University of Toronto has been collecting socio-demographic data from students, faculty and staff through regular surveys, but there is a lack of awareness among the research and innovation community about the University’s data holdings and who can access the data and for what purposes. In addition to Institutional demographic surveys, multiple units collect socio-demographic data on an ad hoc basis.
To support equity diversity and inclusion in research and innovation, this Guide provides an overview of key considerations and best practices for socio-demographic data collection, highlighting University and provincial policy.
Each stage in the data collection process is reviewed including accessing, collecting, managing and reporting socio-demographic data for program evaluation purposes.
This guide is intended for:
Programs and major initiatives that have an institutional commitment to Equity, Diversity and Inclusion in the University of Toronto research environment that seek to:
- Better understand participants, staff, faculty, etc. (group representation).
- Improve equitable delivery of services or programs.
- Identify any biases or systemic barriers to access.
- Plan a targeted program.
- Meet reporting requirements of internal or external stakeholders.
- Measure impact of initiatives (e.g., initiatives to increase diversity).
- Help ensure fairness is embedded across decisions.
- Develop and implement EDI initiatives in a strategic and intentional manner.
This guide is not intended for:
- Guiding data management planning for research projects.
- Collecting, informing, and analyzing data for research queries.
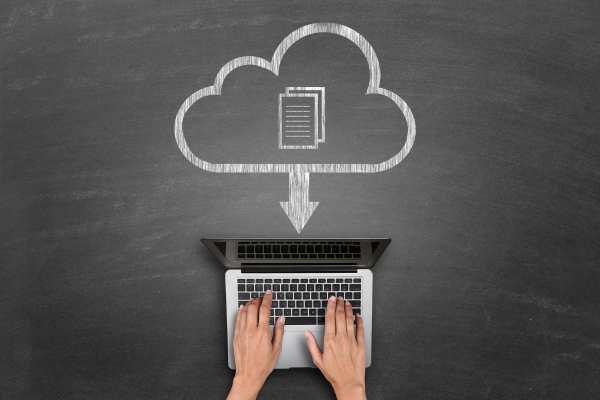
Case Study 1: Coming soon
Case Study 2: Coming soon
Guiding Principles
The Ontario Human Rights Commission (OHRC) supports the collection of data based on code grounds (socio-demographics) as data are a useful and often essential role in supporting human rights and informing human resources strategies. Although necessary, socio-demographic data are also considered sensitive. The sensitive nature of EDI data collection requires adherence to the following principles:
- Respondent privacy and confidentiality are a priority. The University of Toronto is committed to the protection of privacy and protects personal information consistent with the Freedom of Information and Protection of Privacy Act (FIPPA).
- Collect or use data only if needed for established functions. Collect the minimum amount of personal information needed to accomplish the function.
- Minimize respondent burden. When possible, use existing EDI data; if data collection is necessary, only ask questions vital to the program/project’s objectives.
- Ensure the project has the support of senior management or appropriate administrative body.
- Ensure the project has engaged appropriately with communities regarding data collection, analysis, and use.
- Familiarize yourself with the University of Toronto’s Institutional Data Governance Guiding Principles
Although intended for research, many of the principles in the Tri-Council’s Policy Statement: Ethical Conduct for Research Involving Humans framework apply to socio-demographic data for program evaluation. It is beneficial to familiarize yourself with these principles.
Everyone who works with, or has access to, socio-demographic data should consider completing the Tri-Council Policy Statement: Ethical Conduct for Research Involving Humans (TCPS 2) Course so they understand their role and responsibilities in safekeeping personal information.
Collecting Socio-Demographic Data
Identify your team
Identify team members who will be responsible for making decisions and who will be leading the work.
Socio-demographic data can be complex and difficult to interpret. Working with multi-response questions, for example, requires an understanding of data analysis. If possible, ensure you have someone on your team who is trained in relevant competencies such as data collection and data analysis. If this is not possible, it is a good idea to consult with an experienced analyst.
Limit the number of individuals who have access to the data.
Data collection and analysis is not a neutral process. Learn about how unconscious bias can impact the collection, use and reporting of socio-demographic data.
For an introduction to unconscious bias, you may visit the Toronto Initiative for Diversity & Excellence (TID) to access their Unconscious Bias Education Modules.
Define your purpose and objectives
If you need socio-demographic data, your first step should be to clearly define your purpose, objectives and outcomes. Ideally, all team members should understand the purpose and objectives of collecting socio-demographic data.
Answer the following questions:
- What is the purpose of the data?
- What are your desired outcomes of this project?
- How are you going to use the data?
- What resources are available to you to undertake this project?
- Is there someone who can invest the necessary time to manage this project
- Do any staff have knowledge of FIPPA policies and expertise in working with data?
Identify the data you need to meet your purpose
Socio-demographic data capture various dimensions of a person’s identity. The Ontario Human Rights Code prohibits actions that discriminate against people based on protected grounds. The grounds protected under the Code are listed below. This list may help identify the socio-demographic attributes you may want or need to collect.
- Age
- Ancestry, race
- Citizenship
- Ethnic origin
- Place of origin
- Creed
- Disability
- Family status
- Marital status
- Gender identity, gender expression
- Receipt of public assistance (in housing only)
- Record of offences (in employment only)
- Sex (including pregnancy and breastfeeding)
- Sexual orientation.
Balance respecting your participants’ time by collecting the minimum amount of personal information needed to achieve your objectives with the need to capture information for your current and potential future needs.
Government agencies typically focus on the collection of the following dimensions of a person’s identity:
- Gender
- Sexual orientation
- Race/ethnicity
- Indigeneity
- Disability
- Age
See TIPS Questionnaire as an example.
For your purposes, you may also want to consider the following:
- Socio-economic status
- Religion/faith
- Canadian citizenship status
- Residency (Canada, US, outside Canada/US)
In addition to someone’s demographic information, other data may be useful to understand inclusion, barriers, privileges, and opportunities. Examples of these categories are:
- Role (e.g., student, faculty, staff, Principal Investigator, co-investigators, fellows)
- Status (if faculty members)
- Affiliation (e.g., institution, department, Faculty)
- Years of experience (e.g. # of years of independent research, year of study)
Evolving socio-demographic constructs
To compare populations over time, maintaining the measures and terms used for items is important. The University of Toronto provides a list of definitions for EDI items that you may want to consider prior to developing or using other items.
Identify your population
Your identified population for EDI demographic data collection needs to be clearly defined and to be linked to your purpose. Be specific.
Questions to ask yourself:
- Are the participants affiliated with the University of Toronto? What is their role – student, faculty, staff? The data available depends on whether your population comprises University of Toronto students, faculty, and/or staff.
- What programs do I need to include (from one-hour sessions to weekly recurring sessions)?
- What is the timeframe – e.g., past year (fiscal, academic, reporting), snapshot (current), ten years, three months?
- Do participants need to participate for the duration of your timeframe or only at a point in your timeframe?
Create a data management plan
Data management plans (DMP) help data creators and users understand the sequencing of decisions made when creating and utilizing a data set. The plan ensures that data are appropriately managed. A data management plan outlines how data are controlled during the project and after the project is complete and becomes a useful reference tool when new members join the data team.
A data management plan is customized to your project. The details of a data management plan for a new data collection project are different from the details of a plan for the use of secondary data. Either way, it is beneficial to take the time to create a data management plan and update it as changes to your plan are made throughout the life of your project.
Common elements of a data management plan include:
- Purpose of data collection
- Description of data
- Procedures for data collection and/or acquisition
- Definitions of data ownership, access controls and data management procedures
- Procedures for reporting data including privacy and confidentiality
- Data storage and preservation plans
- Accountability for data quality
- Data sharing plans
- Ethics – respecting privacy, obtaining informed consent, ensuring data security
- Special notes about sensitive data
The University of Toronto provides data management guidance and a DMP template.
Population: only U of T participants
If everyone in your target population is affiliated with the University of Toronto, you may not need to collect your own data. The University of Toronto routinely collects socio-demographic data from undergraduate and graduate students, faculty and staff members for internal planning and external reporting purposes.
The student and employment dashboards are published yearly. The student dashboard provides aggregated data for all U of T students or disaggregated by level of instruction (undergraduate/graduate), campus and domestic/international. The Employment Equity Dashboard provides aggregated data staff or disaggregated by campus and employee type (faculty, librarian, staff).
If you require data not available through the dashboards, please contact the stewards of the appropriate datasets.
- Undergraduate student data: data.ovps@utoronto.ca
- Graduate student data: sgs.data@utoronto.ca
- Faculty and staff data: HR Service Centre
To understand whether U of T institutional data is appropriate for your needs, ask yourself:
- Do I have a U of T unique identifier for participants? (e.g., UTORID, student number)
- Does the timeframe match my population’s timeframe?
- Do the attributes I want to include in my analysis exist in the U of T data? (e.g., gender, sexual orientation, etc.) (see student and faculty/staff questionnaires)
- Do the response categories in the U of T data align with my reporting needs? (see student and faculty/staff questionnaires)
- Is my population large enough to receive meaningful aggregated data?
- You may need data at the end of a program, calendar year, fiscal year. Do my reporting and analyses align with the timeframe for reporting U of T data?
- Will aggregated data allow me to conduct the analyses I need? (Individual-level data requests will be approved on a case-by-case basis. If you have answered ‘yes’ to all questions above and require individual-level data, you may be recommended to submit your request via the University’s Common Review Process with the assistance of the appropriate data stewards).
Population: non-U of T participants
If none of your participants are affiliated with the University of Toronto, you will likely have to collect the data.
Collecting sensitive information can cause participants to feel anxiety, distrust and concern about data use, privacy and confidentiality.
To mitigate these concerns:
- Design a process that ensures privacy. Outline how the information collected will be handled and stored confidentially in compliance with privacy, human rights, and other applicable legislation.
- If appropriate, consult with affected communities and other appropriate individuals/organizations.
- Clearly communicate the rationale, method and benefits of collecting data.
Select the data collection tool
Many online survey tools exist. If you are affiliated with U of T, we recommend REDCap.
REDCap is made available to the University of Toronto research community by a partnership between Information Technology Services and the Faculty of Kinesiology and Physical Education, sponsored by the Division of the Vice-President, Research and Innovation.
The Centre for Research and Innovation Support offers REDCap Resources.
Other online data collection tools include:
- SurveyMonkey
- Qualtrics
Notice of collection
All questionnaires need to begin with a notice of collection/consent which should include:
- The purpose for the data collection.
- How much time is required to participate (approximately).
- Confidentiality procedures – reporting, storing data and who will have access.
- Intention to link to other data (if applicable)
- A contact email address respondents can use to ask questions about the questionnaire.
For more information and a template for notices of collection visit UData.
Design the questionnaire
Before creating your own questionnaire, consider the following:
- Using an existing questionnaire (e.g., TIPS, U of T Employment Equity Survey) will allow you to make comparisons to other populations by asking the same questions that other U of T surveys employ
- You will need to use the same questions each time you use the survey if you want to show changes in your program over time. (e.g., time 1 vs. time 2)
Screening/Eligibility
To ensure those who respond to your questionnaire are in your population frame and eligible to respond, include a screening question at the start. At times, you may require more than one question.
Examples of screening/eligibility questions include:
- Student enrolment status (If you are surveying current students, you will want to ensure you don’t inadvertently include students who recently graduated or are not currently enrolled).
- Program attendance (You may want to verify at the start of the questionnaire if the respondent attended the program of interest during the time of interest).
Inclusive language
Ensure you use current wording when writing the questions and response items. Consider the wording of questions to ensure they do not (intentionally or unintentionally) exclude some groups of people. Talk to colleagues who have expertise in the area. You may also want to pilot the survey with a small group.
You may want to consult with appropriate communities to ensure you’re using up-to-date terminology.
Forcing a response / providing ‘prefer not to answer’ option
Online survey tools allow you to ‘force’ a response for each question which means a respondent cannot proceed to the next question without answering the question. If you choose this option, respondents should be given a “prefer not to answer” option for individual questions. Otherwise, do not select the option to force a response in your online survey tool.
Select one or select all that apply
Some questions must be formatted as a multi-select question (where respondents can select more than one response category). One such example is when you are asking respondents to describe their race/ethnicity. Many respondents will identify with more than one race/ethnicity; and this should be reflected in the items on the survey by allowing respondents to select more than one category.
With other questions, such as gender, most respondents will identify with a singular response; however, some may not. In these cases, you will need to weigh the benefits of making the question single or multi-response.
A single response question will lend itself to more straightforward analyses, with participants being sorted into mutually exclusive groups based on their singular selection. There is a risk that respondents may not see themselves represented in the response options.
To allow participants to select more than one gender identity if appropriate, use a multi-response option. Keep in mind this will result in more complex and complicated analyses. This option also necessitates more complex decision processes for the analyst. Will a participant who selected both “woman” and “nonbinary,” be grouped with other women or with other nonbinary participants, or will this participant be represented in both groups? Will the analyst differentiate between women who only selected “woman” as their singular gender identity from those who selected another gender identity in addition to “woman”?
The key is to be intentional, in advance, if possible, about how the analyst will group participants so that you can ask questions and provide response options that will make sense for your purpose and for your participants.
Open-ended questions, write-in boxes & coding
Open-ended questions have the benefit of allowing participants to describe themselves using whatever language they regard as most relevant and accurate to them. However, with open-ended questions, there’s a risk that the data will be uninterpretable and therefore, not unusable. To summarize open-ended questions numerically requires categorization. Coding open-ended responses is very resource intensive. There is a risk coders mis-categorizing responses or apply coding rules inconsistently.
Race/ethnicity
Race/ethnicity are complicated concepts and are difficult to categorize. The Canadian census and other surveys often mix up skin colour and geographic location. The categories in the 2021 census, for example, were: White South Asian (e.g., East Indian, Pakistani, Sri Lankan) Chinese Black Filipino Arab Latin American Southeast Asian (e.g., Vietnamese, Cambodian, Laotian, Thai) West Asian (e.g., Iranian, Afghan) Korean Japanese,
One approach to this issue is to add a preamble to the question acknowledging the difficulties of this question and why you have chosen the response options.
For example, “The terms below reflect terms used in the Canadian census. Using terminology consistent with the census will help us understand our faculty in relation to Canadian demographics.”
Mixed ethnicity
Some race/ethnicity questions offer a ‘mixed ethnicity’ option. This category is broad and difficult to interpret. Instead, keeping the race/ethnicity question as a multi-response without a ‘mixed ethnicity’ option allows you to create a ‘mixed ethnicity’ category once you have collected the data.
Design, program and test questionnaire
Once you have designed the questionnaire including screening and socio-demographic questions, you need to program it into a survey tool.
Before distributing your survey, test it multiple times. Ask colleagues to pilot test the survey.
You want to ensure:
- There are no spelling mistakes or typos.
- All questions and response categories are included.
- Questions are correctly assigned as single or multi-response.
- Questions are correctly assigned as mandatory or not.
- There are no errors in branching logic.
Launch questionnaire
Create a distribution list of everyone in your population which includes:
- Email address or other contact method
- Any other information you may require (e.g., student numbers)
Draft an invitation message.
- Keep it short, simple and compelling.
- Include a contact email for questions.
- Consider who the message will come from
- Include a call to action – (e.g., “please complete”) in the subject line.
- Refer participants to the Notice of Collection for more information.
Anonymous link vs. sending invitations through survey tool
If using an online survey tool such as REDCap, you have the option of sending out invitations through the tool or sending an anonymous link.
If sending an anonymous link, you cannot track who has responded, so you will need to send reminders to everyone in your invitation list.
In addition, with an anonymous link, respondents can respond to the survey multiple times. As an analyst, you do not have a way of knowing if this is the case.
If sending out invitations through the online survey tool, you will need to upload your email addresses to the tool. This will link the responses to email addresses.
Ensure email addresses are deleted from the data file once you have downloaded it and saved the file to a password protected folder.
Once you are certain you have a safe copy of the data file, delete the data from the online tool.
Reminders
You want to find a balance between ensuring everyone in your population has received your invitation and sending too many reminder emails. Often, it takes 3 or 4 attempts.
Population: Combination of U of T and non- U of T participants
Determine whether you will collect new data from all participants
If you have determined that U of T institutional data meet your needs, you have two options:
- Collect data only for those who are not affiliated with U of T, or
- Collect data from all participants.
Consider the benefits of each approach and determine which is best for you.
The strengths of using a hybrid approach:
- Limits survey fatigue among U of T participants.
- Response rate in the institutional data may be higher than what you could achieve through a separate survey.
If you proceed with a hybrid approach, you need to ensure the questions and response items you use to collect new data align with the U of T data so that you can merge the two data sets and/or analyze and report the two data sets.
The strengths of collecting your own data for all U of T and non-U of T participants:
- You have more control over how you ask the questions. You may want to use a standard government form, such as the TIPS self-identification form, or include data that are specifically relevant to your goals and objectives.
Data Preparation
Download data
Depending on the data collection tool you use, you may download your data to Microsoft Excel or a quantitative data analysis program such as R or SPSS.
Microsoft Excel is often the most accessible program; however; it has limitations when it comes to data analysis. Most notably, it is very difficult to analyze multi-response questions, like race/ethnicity, in Excel.
Once you download the data file in the format you need, strip any identifying information, such as a name or email address, save it as “RAW DATA” on a secure server. In addition, password-protect the individual file for added security. Only the analyst(s) should have the password to the file.
Once you have completed the analysis for this file, delete the data from your online survey tool in accordance with your data management plan.
The data should be downloaded and stored on university central or department servers. Do not store data on local drives.
Data Cleaning
Before you begin analysis, you want to copy the RAW DATA file and save it under another name with a different password. You can begin to clean this file and keep the RAW DATA file untouched. You want to check for:
- Qualified respondents – delete non-qualified respondents. At times, you may have respondents who are in the downloaded data but don’t click on the right screening/eligibility questions and are therefore not in the population frame. For example, if you are conducting a survey of current U of T students, but when asked, they say they are not currently a U of T student, you will want to exclude them from the analysis (delete responses).
- Completeness - will you keep responses if a respondent didn’t complete the survey? Some analysts choose to keep responses only if the respondent has answered all the questions. Often this is unnecessary. It is better to have at least some data for your participants than none.
- Invalid responses – Remove “N/A” and inappropriate responses from open-ended questions and questions marked “other, please specify”.
Upcoding
When an ‘other’ category exists in the response categories, either the data need to be placed in an existing response option, or a new response category should be created (if there are enough responses to create a new category). Otherwise, consider keeping an ‘other’ response option.
Address low counts and confidentiality
Confidentiality (minimum n)
Demographic data are personal and therefore confidentiality is of the utmost importance. The minimum cell counts reported to maintain confidentiality is contextual. As a rule, typically categories with fewer than 5 respondents should not be reported. In some cases, 5 is too low a threshold. You need to understand the size of your program, number of participants and the audience for the reported data. There is no one number that will guarantee confidentiality. To be safe, ask yourself if any individuals can be identified?
Recoding for privacy
Sometimes, when a socio-demographic question has only one or a few responses for a particular category, the respondent may become identifiable. When response options have a small number of respondents (n≤5), they should be re-coded, if possible, into an existing category, or a new category (such as ‘other’) should be created that includes 5 or more respondents. If not possible, you should indicate that the frequency is too low to report.
What to do when numbers are too low
Often, programs do include a small number of participants which makes it difficult to analyze the data. Other times, even though programs have large enough participants, they may include very few participants from specific attributes. Regardless of the reason, low numbers are problematic for two reasons:
- Data can be unreliable, and results do not offer any insight.
- Confidentiality of participants cannot be maintained.
An effort should be made to increase the size of the numerator by collapsing data categories or combining years/sessions.
Collapsing data categories
Although not ideal, because of low counts in some categories, sometimes you will have to collapse some categories. For example, if you have the following categories for gender and ‘Gender fluid’, ‘non-binary’, ‘Two-spirit’ and ‘questioning’, have very few or no responses, you may need to collapse those categories and show responses for ‘Man’, ‘Woman’ and ‘Another category’.
- Gender fluid
- Man
- Non-binary
- Two-spirit
- Woman
- Questioning
Combining years/sessions
You may have to collapse years or sessions of the program. Sometimes this will work for your analysis but other times it may not. For example, if you have implemented a new initiative, combining years before and after the initiative will not allow you to understand if the initiative has made an impact.
Alternative methods
When participant numbers are low, you may have to pursue options other than a survey.
Qualitative research is often a good option. Data from qualitative research (e.g., interviews or focus groups) may not provide answers to your original questions, but they can provide insight into participants’ lived experiences and complex narratives that can be helpful in reviewing your programs.
Data Analysis
Types of Analysis
Quantitative data analysis does not need to be complicated. Often, descriptives (i.e., frequencies or percentages) are all you need, but the type of analyses will depend on your purpose and objectives.
Frequencies
Frequencies tell you how many times a particular response option was chosen. (e.g., the number of respondents who selected the ‘woman’ gender category).
Percentages
Percentages describe the information as a proportion of the whole. The whole is the number of respondents for a particular question. Percentages are more meaningful than frequencies when comparing groups of respondents or categories. (e.g., instead of reporting that “30 respondents selected ‘woman’”, it is more meaningful to report that “60% of respondents identify as ‘women’”.
If you have a small sample size, percentages can be misleading, therefore, include the counts.
Mean and median
Mean and median are measures of central tendency and are used with continuous data (e.g., age).
Most socio-demographic data is categorical data and as such do not have a mean or median. You may find it useful to use means and/or medians for variables such as age, number of years living in Canada, years since student, faculty, etc.
Individual Attributes vs. Intersectionality
Often, socio-demographic data is presented by individual attributes (e.g., gender, sexual orientation). The intersectionality of an individual’s identities is a key component of understanding equity and barriers to inclusion and equity.
In addition to reporting on topline socio-demographic data it is necessary to look at intersectional identities using crosstabulations. For example, you may want to report race/ethnicity within gender, or sexual orientation within gender, or disability within age group.
Linking data
Often, collecting socio-demographic data alone is not enough to meet your information needs. You may need to link these data to other information you have about your participants. If for example, you are using U of T institutional data and you would like to link them to data you have about your participants (e.g., grant application success rate), you will need to use a unique identifier to link U of T data, with your data. In this case, you would need to request individual-level data in the common review so that you can link the two datasets using a unique identifier (e.g., student number or UTORID).
You may be interested in understanding how individuals from different backgrounds experience your programs (e.g., feelings of inclusion), If you are conducting a survey about experiences, and you are collecting socio-demographics data, you do not have to link two datasets. If, for some reason (see Acceleration Consortium case for example), you are collecting your own socio-demographic data at a different time than experience data, you would need to have a unique identifier in both datasets so you can link responses to individuals.
Sharing Data
You may need to report the data to the program/institution lead, internal or external stakeholders or the public at large.
The confidentiality of your participants is sacrosanct.
Aggregate data
You may need to create different reports for your various audiences. Most audiences only require aggregated data. Consider using Microsoft Word, Excel or if you have much data to report, and have knowledge of Power BI or Tableau, you may use these data visualization tools.
Include with data reporting:
- Data source
- Number of respondents
- Response rate if applicable
Individual-level data
You may not share individual-level data. Only individuals on your team who will analyze and/or prepare reports may have access to individual-level data. Keep the number of individuals to a minimum and observe the ‘need to know’ principle. Ask yourself, “does this individual need to have access to individual-level data’ to perform their duties?”.
Team vs. institutional/public reporting
Carefully consider what information is appropriate to share outside of your team. Often, data for program evaluation is most useful to program planners, leads and the executive team and not necessarily appropriate for external audiences.
Storing and Disposing of Data
Storage and disposal procedures should be outlined in your data management plan including length of data storage and disposal procedures. The plan should be reviewed regularly to ensure the details remain relevant.
Given the sensitive nature of socio-demographic data, you must consider the risks of harm if the data were to be accessed or disclosed. To protect the privacy of participants and to reduce potential harm to individuals and communities all efforts must be taken to ensure the data are not exposed to unauthorized access.
- Data should be kept for at least one year. The length of time depends on several factors, including project goals and objectives and your unit’s policies and procedures.
- Data should be stored in password-protected servers.
- The data file should also be password protected.
- The data should be de-identified (e.g., not include names, email addresses).
- Data should not be stored on local file system or local database.
- To identify secure data storage options, units are encouraged to connect with local IT groups or ITS. https://its.utoronto.ca/
You may contact UTARMS for assistance in determining retention and disposition decisions specific to your institutional data. They will ask to see your data management plan.
The Freedom of Information and Protection of Privacy Office offers guidelines and best practices resources that will help guide you through this process.
Consultations
The CRIS Special Projects Officer, EDI, is piloting a coaching service for faculty and their research teams in the planning and execution of collecting, managing and reporting EDI data for program evaluation purposes.
Have a need for EDI data you would like our help with or just want to learn more about this support?
Submit your request here and a member of our team will be in touch to book your initial consultation!
We will work with you to:
- Refine project goals, identify a population frame, and develop an appropriate project design
- Follow data privacy and confidentiality protocols
- Make choices about the data to collect and how to collect it
- Navigate the University of Toronto’s Common Review process
- Develop a questionnaire that best meets the data needs of the project (question wording, positioning, response type)
- Program your questionnaire in REDCap or other online survey tool
- Plan data analysis and reporting.
If you have any questions about this guide please contact us at cris@utoronto.ca.